Do models need to explain?
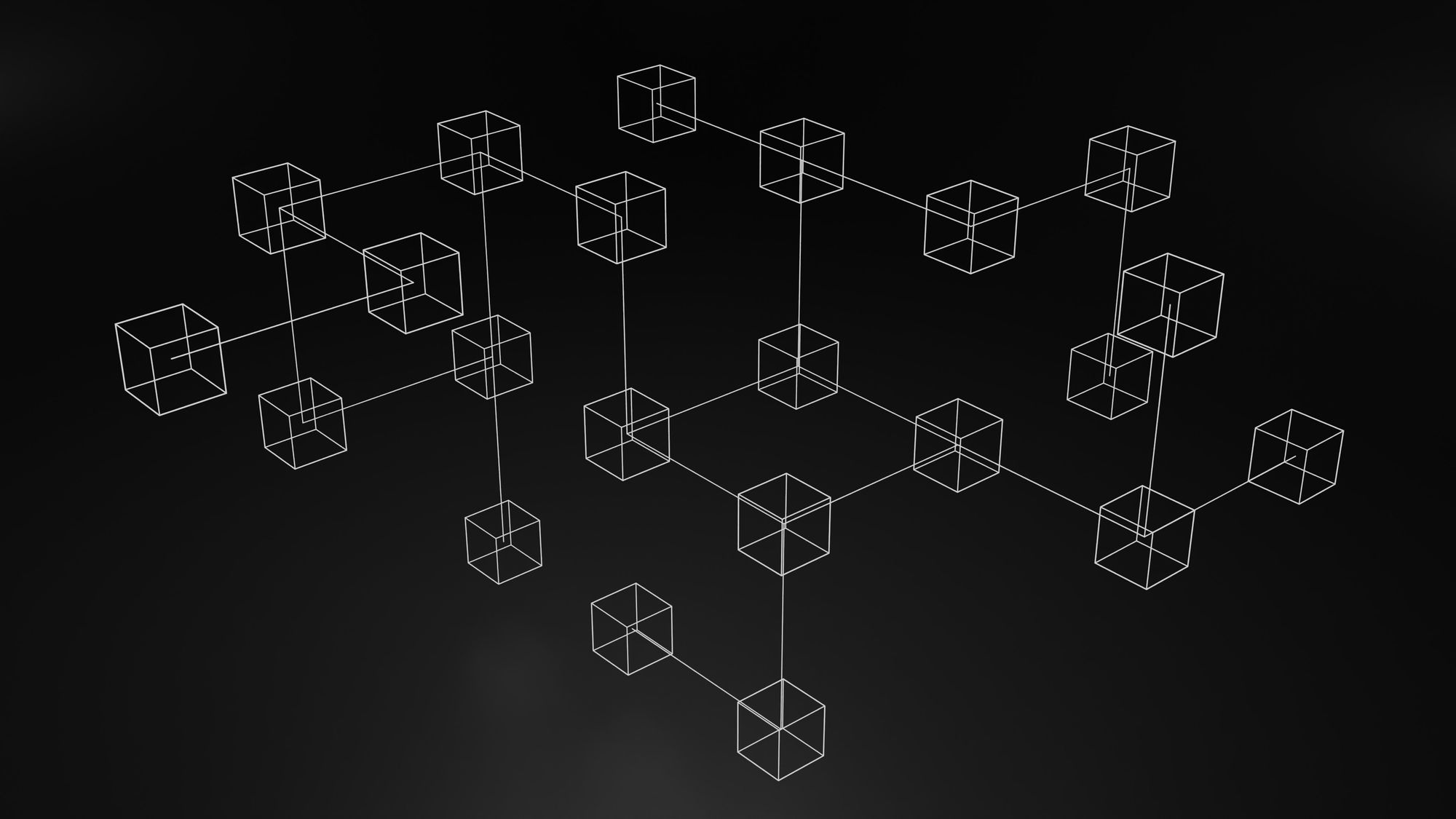
Models are constructs of reality. They condense knowledge in order to help us understand reality and support our ideas.
One way that we can test the validity of a model is to perform an experiment. For instance, if a model accurately predicts an experiment outcome, it is verified. But if a model makes a false prediction, it signals that the model is flawed and needs to be revised.
The way you build and use a model depends on your choice of experimental framework. I've previously discussed such frameworks here.
Under the hypothesis framework, models are built on explanatory theories. Whereas models built with the question framework rely exclusively upon predictions. Let's consider how models fit into these frameworks a little further.
Models aren't a central part of the hypothesis framework. This is because this approach recognizes that the primary aim of science is explanation. And so experiments are tools by which we subject hypotheses to falsification. They are not used to confirm our predictions. Because of this focus on error correction, there is little need to construct models for verification — though they may help us to communicate or comprehend complex mechanisms.
Even though hypothesis testing focuses on falsification of explanatory theories, it doesn't mean that prediction and reproducability are unimportant. It's just that the hypothesis framework views prediction as supplementary to explanation. Of course, our best theories explain the world and make accurate predictions at the same time. And it's also vital that we can reproduce experimental results. We shouldn't confuse the need for explanatory theories with a disregard for reproducability.
Critically, models built under the hypothesis framework consist of explanatory theories.
On the other hand, models are central to the question framework. With this method, one gathers data in response to a question and builds a model from it. This model is then verified by testing its predictions. Because it is based on inductivism, the model is built solely on observations. Yes, the model is explanationless.
Explanationless models are problematic. The idea that theories or models needn't do more than make predictions is called instrumentalism. In this view, the only way in which we can determine the truth of a theory is the extent to which it predicts experiment outcomes. This position opposes realism — the true idea that scientific theories can reliably predict and explain reality.
Some may argue it's superfluous to ask models or theories to explain when they can adequately predict. For practical matters, our ability to say that a certain treatment will reliably save someones life, for example, is arguably all that matters. Do we really need to understand the why? I think we must.
Keeping with the life-saving treatment example, if we can explain how a certain treatment works, then we have a clear rationale to test it in other situations, or even try to develop other treatments with greater efficacy and less side effects, since we can explain its mode of action. On what grounds could we do this otherwise? We certainly wouldn't be justified to do so on prediction alone.
“There is nothing more practical than a good theory” (Lewin, 1952)
Another issue with explanationless models relates to verification. Let's imagine that we've tested a model built from our prior data. And the experiment outcome conflicts with the predictions made by our model. What would we do next?
According to the approach, we would simply change the model. But how exactly? The model we made was built on prior observations. There is a dilemma here. And it speaks to a critique of inductivism — the future does not always resemble the past.
This is why we need models consisting of explanatory theories. For they account for our observations, which by themselves, tell us nothing.